Summary of Design-Learn (DL) Domain
Sachiyo ABURATANI
Deputy Director
Computational Bio Big-Data Open Innovation Laboratory,
National Institute of Advanced Industrial Science and Technology (AIST)
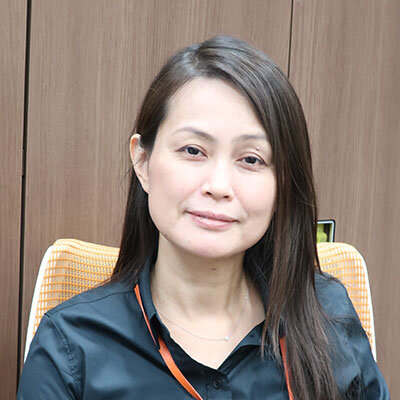

Sachiyo ABURATANI
Deputy Director
Computational Bio Big-Data Open Innovation Laboratory,
National Institute of Advanced Industrial Science and Technology (AIST)
The DBTL cycle in this project means Design (cell design) → Build (host construction) → Test (productivity evaluation) → Learn (learning of results). Of these, the Design (cell design) and Learn (learning of results) steps utilize information analysis technologies.
In the field of business, a method called the PDCA cycle is becoming widely used. The PDCA cycle is a strategic method for continuously improving various operations in business management, by repeatedly running the cycle of Plan (planning) → Do (execution) → Check (evaluation) → Action (improvement). This cyclic improvement method is also being developed as a DBTL cycle in this project, which aims at efficient material production by microorganisms. Of the DBTL cycle in this project [Design (cell design) → Build (host construction) → Test (productivity evaluation) → Learn (learning of results)], the Design (cell design) and Learn (learning of results) steps use information analysis technologies.
Japan has a long history in the field of fermentation and production, and has been ahead of other countries in breeding and modification technology for host microorganisms, etc. However, the field of "bio-based production" using synthetic biology lags behind Europe and the United States. The "bio-based production" conducted in Europe and the United States consists of the acquisition of large-scale data using robotics, the identification of candidate genes for modification using AI technology, such as machine learning and deep learning, and more efficient construction of material production strains through the genetic modification of host cells. In this project, while incorporating the advantages of these concepts but not just simply following other countries' lead, we are developing a smart cell design system as a new information analysis technology to strengthen global competitiveness and activate the "manufacturing industry" in Japan.
The foundation for the information science technology used in host cell modifications, led by Europe and the United States, is machine learning. The advantages of this technology are that a large amount of data (tens of thousands to hundreds of thousands of samples) enables highly accurate rule extraction, and that the accuracy improves as the number of data increases over time. On the other hand, in order to obtain a certain level of accuracy, data as large as about ten-thousands of samples are required for each host cell and production material, raising concern about a greater economic burden. At present, to compete internationally on an equal footing with these leading countries in this field where Japan has fallen behind, it is essential for Japan to overcome the two disadvantages of using machine learning only, namely, "mass data" and "host-dependence." Therefore, in this project, to construct "smart cells, i.e., living cells having highly optimized and artificially designed capabilities to produce highly functional materials," a smart cell design system has been developed, which can be used with [1] a realistic number of data samples (a minimum of about 100 samples), [2] more accurately, and [3] host-independently, by integrating Japan's unique information analysis technologies and superior bioinformatic technologies.
図1.現場課題を情報解析へ
Figure 1 shows the relationship between challenges occurring at the production site and information analysis-based solutions and approaches in the development of this system. Each challenge occurring at the production site is listed on the left. First, in order to realize these challenges with a new concept of living cells called smart cells, the challenges were roughly classified into 4 groups. To overcome these 4 challenges, we are developing the following information analysis technologies.
≫Technology of Knowledge Extraction and Learning from Literature, etc.(Proposing Enzymes Utilizing Machine Learning・Knowledge Base Supporting Smart Cell Designing)
≫Metabolic Design and Optimization Technology
≫Gene Regulatory Network Modeling Technology
≫Gene Sequence Design Technology
≫Enzyme Modification Design Technology
The above information analysis technologies, the theoretical basis for which has already been published by the project participants, have been established as a theoretical system (References 1, 2, 3, and 4). In this project, whose objective is to put into practical use such various, theoretically backed up technologies, we are collaborating with a task demonstration group that has various challenges at the production site. By improving and modifying our developed theoretical base for designing smart cells in each demonstration task and by feeding back the obtained results to the material production site, we have been developing information analysis technologies that can be used more practically.
Finally, Fig. 2 shows the overall configuration of the smart cell design system being developed in this project. This system is structured by setting the above-mentioned database, which has been constructed in this project as the base of the various information analysis technologies, as the hub. In this system, respective information analysis technologies can be applied to data stored in the database. The database, located at the core of the system, stores data for learning, including various data measured in the project and data required to design smart cells obtained from existing databases. By using these publicly known data and our original data in combination, the information analysis technologies listed in the middle circle are applied. By applying the information analysis technologies, various "models" listed in the outer circle are constructed. The "model" derived here means a schematic diagram of a system that simply shows living activities expressed by graphs, pathways, or simulations that represent the functions of living cells. By interpreting the various models constructed in this way, the system has realized the provision of proposals on candidate genes for modification that could not be predicted in the conventional breeding methods, the design of gene sequences to be introduced to host cells, and the provision of proposals on novel biosynthetic pathways that living cells did not originally have.
図2.スマートセル設計システム全体像
1)Araki, M., et al., Bioinformatics, 31(6), 905-911, 2015
2)Shirai, T., et al.,Microbial Cell Factories, 15(13), 1-6, 2016
3)Aburatani, S., Gene regulation and systems biology, 5, 75-88, 2011
4)Kameda, T., et al.,Proc. Natl. Acad. Sci., 103 (47), 17765-17770, 2006
Last updated:December 25, 2023